Generations of engineers and scientist couldn’t let go of the idea of artificial intelligence. The fascination for the subject withstood a lot of challenges but today, A.I. can be found in many areas of our lives. Behind artificial intelligence isn’t just one technique. There are many different techniques and mathematical models. Hence, different terms pop up in relation to A.I. and they can cause quite a lot of confusion. We want to shed some light on a few of those terms.
Artificial intelligence is by no means something new. It all started in the middle of the 20th century. Since then the progress of A.I. faced quite a few setbacks: While there was plenty of curiosity and motivation to do research, for many years, computer technology couldn’t properly meet the needs of the vast amount of necessary processing and storing. Furthermore, funding was withdrawn again and again because the outcomes weren’t exactly as groundbreaking as the sponsors had hoped. So, it took a while for A.I. to become more than theories and science-fiction.
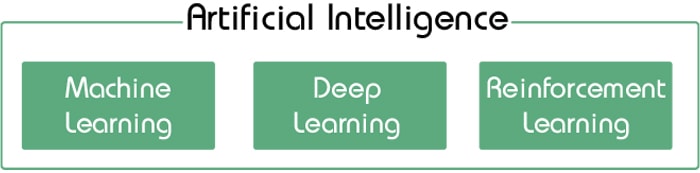
A.I. contains many different models and techniqes. Some of the more well-known are machine learning, deep learning and reinforcement learning.
A.I. – The mothership
The goal of artificial intelligence is basically to mimic human behavior. The technology can perform complex tasks that would need the cognitive abilities of a human. To achieve this, a lot of models and different techniques have been developed. You probably already heard of Machine Learning, Deep Learning and Reinforcement Learning. They all are subfields of A.I.
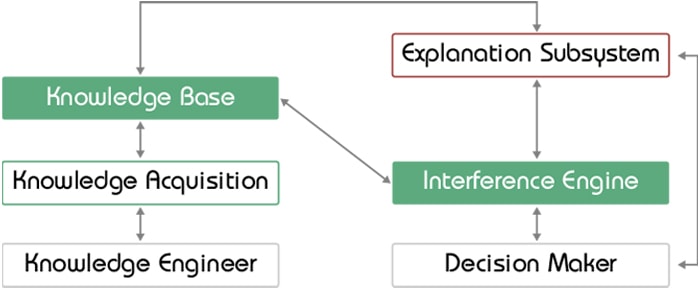
Two of the most crucial components of an expert system are the knowledge base that contains the organized facts, and the interference engine that interprets and evaluates the facts.
GOFAI – Good-old-fashioned A.I.
GOFAI dominated the field until the late 80s. It is based on hard coded algorithms with explicitly programmed if-then statements. GOFAI had its commercial breakthrough in the 80s, when companies saved a lot of money by using expert systems. These systems rely on a knowledge base with facts and a component that interprets these facts. Amongst others an expert system can monitor, classify or plan but all within a very specific field.
The weak and the strong
Where so-called weak A.I. completes tasks without mimicking human reasoning but using for example matching patterns against an input of conditions. Strong A.I. however, mirrors human thinking. The advances of neuroscience in the 1990s gave the not very popular bottom-up app roach and its focus on neural networks a major boost. The difference between GOFAI and Machine Learning is what the name of the latter implies: Learning.
Machine Learning
Machine Learning is a part of the A.I. sphere. It focuses on the question “How do machines learn?”. With ML – short for Machine Learning – computers are imitating how humans learn and therefore they are mimicking how humans make decisions. The mathematical models used in ML need a vast amount of data in order for them to make predictions or find solutions. Two of the techniques of Machine Learning are Deep Learning and Reinforcement Learning.
Deep Learning
The structures of a human brain are the basics for the algorithms used in Deep Learning. Neurons receive signals, process them and pass them on to the other neurons. A Deep Learning algorithm works similarly. It’s build on layers and the complexity of the task determines how deep the network needs to go. These artificial neural networks need a lot of data to learn from. Whatever knowledge they gained can then be applied to a new set of data.
Reinforcement Learning
Reinforcement Learning relies on feedback from the environment. This trial and error technique aims for rewards. Often the go-to example of Reinforcement Learning is a child who learns to walk. By falling down, it adjusts its step and movements. The reward is being able to walk. Since Reinforcement Learning is an independent, self-teaching system it can adapt to its environment if the complexity of the task requires it.
Expect more
Although researchers and engineers already did an incredible job regarding the progress of A.I. and implementing it in things we use in our daily life – like Siri, Spotify, chat bots, drones, … there is still a lot to discover. The techniques already in use to are not exclusive – they can be combined and together with the possibilities that are yet to be discovered there is immense potential to increase efficiencies in every aspect of our lives.